After reading a few books on data science and a little bit about machine learning, I felt it was time to round out my studies in these subjects with a couple more books. I was hoping to get some more exposure to implementing different machine learning algorithms as well as diving deeper into how to effectively use the different Python tools for machine learning, and these two books seemed to fit the bill. The first book with the upside-down face,
Data Smart: Using Data Science to Transform Data Into Insight by John W. Foreman, looked like it would fulfill the former goal and do it all in Excel, oddly enough. The second book with the right side-up face,
Python Machine Learning: Machine Learning and Deep Learning with Python, scikit-learn, and TensorFlow by Sebastian Raschka and Vahid Mirjalili, promised to address the second goal. Let's see how these two books complement each other and move the reader toward a better understanding of machine learning.
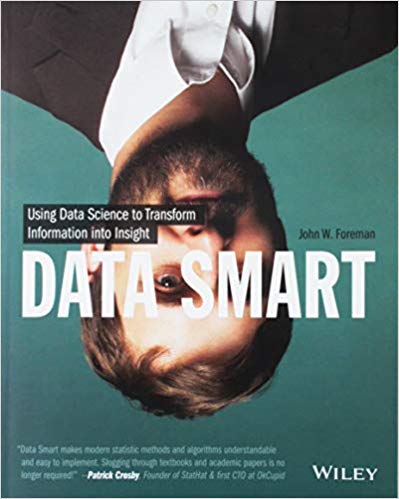 | VS. | 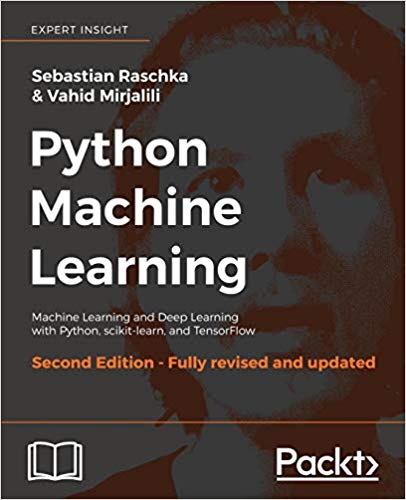 |
Data Smart
I must admit; I was somewhat hesitant to get this book. I was worried that presenting everything in Excel would be a bit too simple to really learn much about data science, but I needn't have been concerned. This book was an excellent read for multiple reasons, not least of which is that Foreman is a highly entertaining writer. His witty quips about everything from middle school dances to Target predicting teen pregnancies were a great motivator to keep me reading along, and more than once I caught myself chuckling out loud at an unexpectedly absurd reference.
It was refreshing to read a book about data science that didn't take itself seriously and added a bit of levity to an otherwise dry (interesting, but dry) subject. Even though it was lighthearted, the book was not a joke. It had an intensity to the material that was surprising given the medium through which it was presented. Spreadsheets turned out to be a great way to show how these algorithms are built up, and you can look through the columns and rows to see how each step of each calculation is performed. Conditional formatting helps guide understanding by highlighting outliers and important contrasts in the rows of data. Excel may not be the best choice for crunching hundreds of thousands of entries in an industrial-scale model, but for learning how those models actually work, I'm convinced that it was a worthy choice.
The book starts out with a little introduction that describes what you got yourself into and justifies the choice of Excel for those of us that were a bit leery. The first chapter gives a quick tour of the important parts of Excel that are going to be used throughout the book—a skim-worthy chapter. The first real chapter jumps into explaining how to build up a k-means cluster model for the highly critical task of grouping people on a middle school dance floor. Like most of the rest of the chapters, this one starts out easy, but ramps up the difficulty so that by the end we're clustering subscribers for email marketing with a dozen or so dimensions to the data.
Chapter 3 switches gears from an unsupervised to a supervised learning model with naïve Bayes for classifying tweets about Mandrill the product vs. the animal vs. the Mega Man X character. Here we can see how irreverent, but on-point Foreman is with his explanations:
Because naïve Bayes is often called "idiot's Bayes." As you'll see, you get to make lots of sloppy, idiotic assumptions about your data, and it still works! It's like the splatter-paint of AI models, and because it's so simple and easy to implement (it can be done in 50 lines of code), companies use it all the time for simple classification jobs.
Every chapter is like this and better. You never know what Foreman's going to say next, but you quickly expect it to be entertaining. Case in point, the next chapter is on optimization modeling using an example of, what else, commercial-scale orange juice mixing. It's just wild; you can't make this stuff up. Well, Foreman can make it up, it seems. The examples weren't just whimsical and funny, they were solid examples that built up throughout the chapter to show multiple levels of complexity for each model. I was constantly impressed with the instructional value of these examples, and how working through them really helped in understanding what to look for to improve the model and how to make it work.
After optimization came another dive into cluster analysis, but this time using network graphs to analyze wholesale wine purchasing data. This model was new to me, and a fascinating way to use graphs to figure out closely related nodes. The next chapter moved on to regression, both linear and non-linear varieties, and this happens to be the Target-pregnancy example. It was super interesting to see how to conform the purchasing data to a linear model and then run the regression on it to analyze the data. Foreman also had some good advice tucked away in this chapter on data vs. models:
You get more bang for your buck spending your time on selecting good data and features than models. For example, in the problem I outlined in this chapter, you'd be better served testing out possible new features like "customer ceased to buy lunch meat for fear of listeriosis" and making sure your training data was perfect than you would be testing out a neural net on your old training data.
Why? Because the phrase "garbage in, garbage out" has never been more applicable to any field than AI. No AI model is a miracle worker; it can't take terrible data and magically know how to use that data. So do your AI model a favor and give it the best and most creative features you can find.
As I've learned in the other data science books, so much of data analysis is about cleaning and munging the data. Running the model(s) doesn't take much time at all.
We're into chapter 7 now with ensemble models. This technique takes a bunch of simple, crappy models and improves their performance by putting them to a vote. The same pregnancy data was used from the last chapter, but with this different modeling approach, it's a new example. The next chapter introduces forecasting models by attempting to forecast sales for a new business in sword-smithing. This example was exceptionally good at showing the build-up from a simple exponential smoothing model to a trend-corrected model and then to a seasonally-corrected cyclic model all for forecasting sword sales.
The next chapter was on detecting outliers. In this case, the outliers were exceptionally good or exceptionally bad call center employees even though the bad employees didn't fall below any individual firing thresholds on their performance ratings. It was another excellent example to cap off a whole series of very well thought out and well executed examples. There was one more chapter on how to do some of these models in R, but I skipped it. I'm not interested in R, since I would just use Python, and this chapter seemed out of place with all the spreadsheet work in the rest of the book.
What else can I say? This book was awesome. Every example of every model was deep, involved, and appropriate for learning the ins and outs of that particular model. The writing was funny and engaging, and it was clear that Foreman put a ton of thought and energy into this book. I highly recommend it to anyone wanting to learn the inner workings of some of the standard data science models.
Python Machine Learning
This is a fairly long book, certainly longer than most books I've read recently, and a pretty thorough and detailed introduction to machine learning with Python. It's a melding of a couple other good books I've read, containing quite a few machine learning algorithms that are built up from scratch in Python a la
Data Science from Scratch, and showing how to use the same algorithms with scikit-learn and TensorFlow a la the
Python Data Science Handbook. The text is methodical and deliberate, describing each algorithm clearly and carefully, and giving precise explanations for how each algorithm is designed and what their trade-offs and shortcomings are.
As long as you're comfortable with linear algebraic notation, this book is a straightforward read. It's not exactly easy, but it never takes off into the stratosphere with the difficulty level. The authors also assume you already know Python, so they don't waste any time on the language, instead packing the book completely full of machine learning stuff. The shorter first chapter still does the introductory tour of what machine learning is and how to install the correct Python environment and libraries that will be used in the rest of the book. The next chapter kicks us off with our first algorithm, showing how to implement a perceptron classifier as a mathematical model, as Python code, and then using scikit-learn. This basic sequence is followed for most of the algorithms in the book, and it works well to smooth out the reader's understanding of each one. Model performance characteristics, training insights, and decisions about when to use the model are highlighted throughout the chapter.
Chapter 3 delves deeper into perceptrons by looking at different decision functions that can be used for the output of the perceptron model, and how they could be used for more things beyond just labeling each input with a specific class as described here:
In fact, there are many applications where we are not only interested in the predicted class labels, but where the estimation of the class-membership probability is particularly useful (the output of the sigmoid function prior to applying the threshold function). Logistic regression is used in weather forecasting, for example, not only to predict if it will rain on a particular day but also to report the chance of rain. Similarly, logistic regression can be used to predict the chance that a patient has a particular disease given certain symptoms, which is why logistic regression enjoys great popularity in the field of medicine.
The sigmoid function is a fundamental tool in machine learning, and it comes up again and again in the book. Midway through the chapter, they introduce three new algorithms: support vector machines (SVM), decision trees, and K-nearest neighbors. This is the first chapter where we see an odd organization of topics. It seems like the first part of the chapter really belonged with chapter 2, but including it here instead probably balanced chapter length better. Chapter length was quite even throughout the book, and there were several cases like this where topics were spliced and diced between chapters. It didn't hurt the flow much on a complete read-through, but it would likely make going back and finding things more difficult.
The next chapter switches gears and looks at how to generate good training sets with data preprocessing, and how to train a model effectively without overfitting using regularization. Regularization is a way to systematically penalize the model for assigning large weights that would lead to memorizing the training data during training. Another way to avoid overfitting is to use ensemble learning with a model like random forests, which are introduced in this chapter as well. The following chapter looks at how to do dimensionality reduction, both unsupervised with principal component analysis (PCA) and supervised with linear discriminant analysis (LDA).
Chapter 6 comes back to how to train your dragon…I mean model…by tuning the hyperparameters of the model. The hyperparameters are just the settings of the model, like what its decision function is or how fast its learning rate is. It's important during this tuning that you don't pick hyperparameters that are just best at identifying the test set, as the authors explain:
A better way of using the holdout method for model selection is to separate the data into three parts: a training set, a validation set, and a test set. The training set is used to fit the different models, and the performance on the validation set is then used for the model selection. The advantage of having a test set that the model hasn't seen before during the training and model selection steps is that we can obtain a less biased estimate of its ability to generalize to new data.
It seems odd that a separate test set isn't enough, but it's true. Training a machine isn't as simple as it looks. Anyway, the next chapter circles back to ensemble learning with a more detailed look at bagging and boosting. (Machine learning has such creative names for things, doesn't it?) I'll leave the explanations to the book and get on with the review, so the next chapter works through an extended example application to do sentiment analysis of IMDb movie reviews. It's kind of a neat trick, and it uses everything we've learned so far together in one model instead of piecemeal with little stub examples. Chapter 9 continues the example with a little web application for submitting new reviews to the model we trained in the previous chapter. The trained model will predict whether the submitted review is positive or negative. This chapter felt a bit out of place, but it was fine for showing how to use a model in a (semi-)real application.
Chapter 10 covers regression analysis in more depth with single and multiple linear and nonlinear regression. Some of this stuff has been seen in previous chapters, and indeed, the cross-referencing starts to get a bit annoying at this point. Every single time a topic comes up that's covered somewhere else, it gets a reference with the full section name attached. I'm not sure how I feel about this in general. It's nice to be reminded of things that you've read about hundreds of pages back and I've read books that are more confusing for not having done enough of this linking, but it does get tedious when the immediately preceding sections are referenced repeatedly. The next chapter is similar with a deeper look at unsupervised clustering algorithms. The new k-means algorithm is introduced, but it's compared against algorithms covered in chapter 3. This chapter also covers how we can decide if the number of clusters chosen is appropriate for the data, something that's not so easy for high-dimensional data.
Now that we're two-thirds of the way through the book, we come to the elephant in the machine learning room, the multilayer artificial neural network. These networks are built up from perceptrons with various activation functions:
However, logistic activation functions can be problematic if we have highly negative input since the output of the sigmoid function would be close to zero in this case. If the sigmoid function returns output that are close to zero, the neural network would learn very slowly and it becomes more likely that it gets trapped in the local minima during training. This is why people often prefer a hyperbolic tangent as an activation function in hidden layers.
And they're trained with various types of back-propagation. Chapter 12 shows how to implement neural networks from scratch, and chapter 13 shows how to do it with TensorFlow, where the network can end up running on the graphics card supercomputer inside your PC. Since TensorFlow is a complex beast, chapter 14 gets into the nitty gritty details of what all the pieces of code do for implementation of the handwritten digit identifier we saw in the last chapter. This is all very cool stuff, and after learning a bit about how to do the CUDA programming that's behind this library with
CUDA by Example, I have a decent appreciation for what Google has done with making it as flexible, performant, and user-friendly as they can. It's not simple by any means, but it's as complex as it needs to be. Probably.
The last two chapters look at two more types of neural networks: the deep convolutional neural network (CNN) and the recurrent neural network (RNN). The CNN does the same hand-written digit classification as before, but of course does it better. The RNN is a network that's used for sequential and time-series data, and in this case, it was used in two examples. The first example was another implementation of the sentiment analyzer for IMDb movie reviews, and it ended up performing similarly to the regression classifier that we used back in chapter 8. The second example was for how to train an RNN with Shakespeare's
Hamlet to generate similar text. It sounds cool, but frankly, it was pretty disappointing for the last example of the most complicated network in a machine learning book. It generated mostly garbage and was just a let-down at the end of the book.
Even though this book had a few issues, like tedious code duplication and explanations in places, the annoying cross-referencing, and the out-of-place chapter 9, it was a solid book on machine learning. I got a ton out of going through the implementations of each of the machine learning algorithms, and wherever the topics started to stray into more in-depth material, the authors provided references to the papers and textbooks that contained the necessary details.
Python Machine Learning is a solid introductory text on the fundamental machine learning algorithms, both in how they work mathematically how they're implemented in Python, and how to use them with scikit-learn and TensorFlow.
Of these two books,
Data Smart is a definite-read if you're at all interested in data science. It does a great job of showing how the basic data analysis algorithms work using the surprisingly effect method of laying out all of the calculations in spreadsheets, and doing it with good humor.
Python Machine Learning is also worth a look if you want to delve into machine learning models, see how they would be implemented in Python, and learn how to use those same models effectively with scikit-learn and TensorFlow. It may not be the best book on the topic, but it's a solid entry and covers quite a lot of material thoroughly. I was happy with how it rounded out my knowledge of machine learning.